Artificial Representation: pitfalls at the intersection of AI and DEI
Images are powerful. And almost nothing grabs attention or is as effective of a tool for connection as a compelling photo or video of a fellow human being.
But in the nonprofit sector, where budgets are low and time is precious, a good photo (or video clip) can be challenging to source. Using images comes with additional parameters around representation, consent, and more, if your organization upholds standards around using images in equitable ways, and that center diversity and inclusion. And in this very specific nonprofit context of visual scarcity and ethical constraints, AI has entered the picture.
AI is abundant. AI is prolific. AI can create mission-specific visuals, on demand. And, AI is affordable (for now). And so it’s no surprise that more and more, AI-generated content is showing up in stock photo offerings, and in the brands and communications that use those resources. For example, here are 3 images from the first page of results when we searched “LGBTQ Youth” in Adobe stock recently—can you spot which photo is AI-generated?
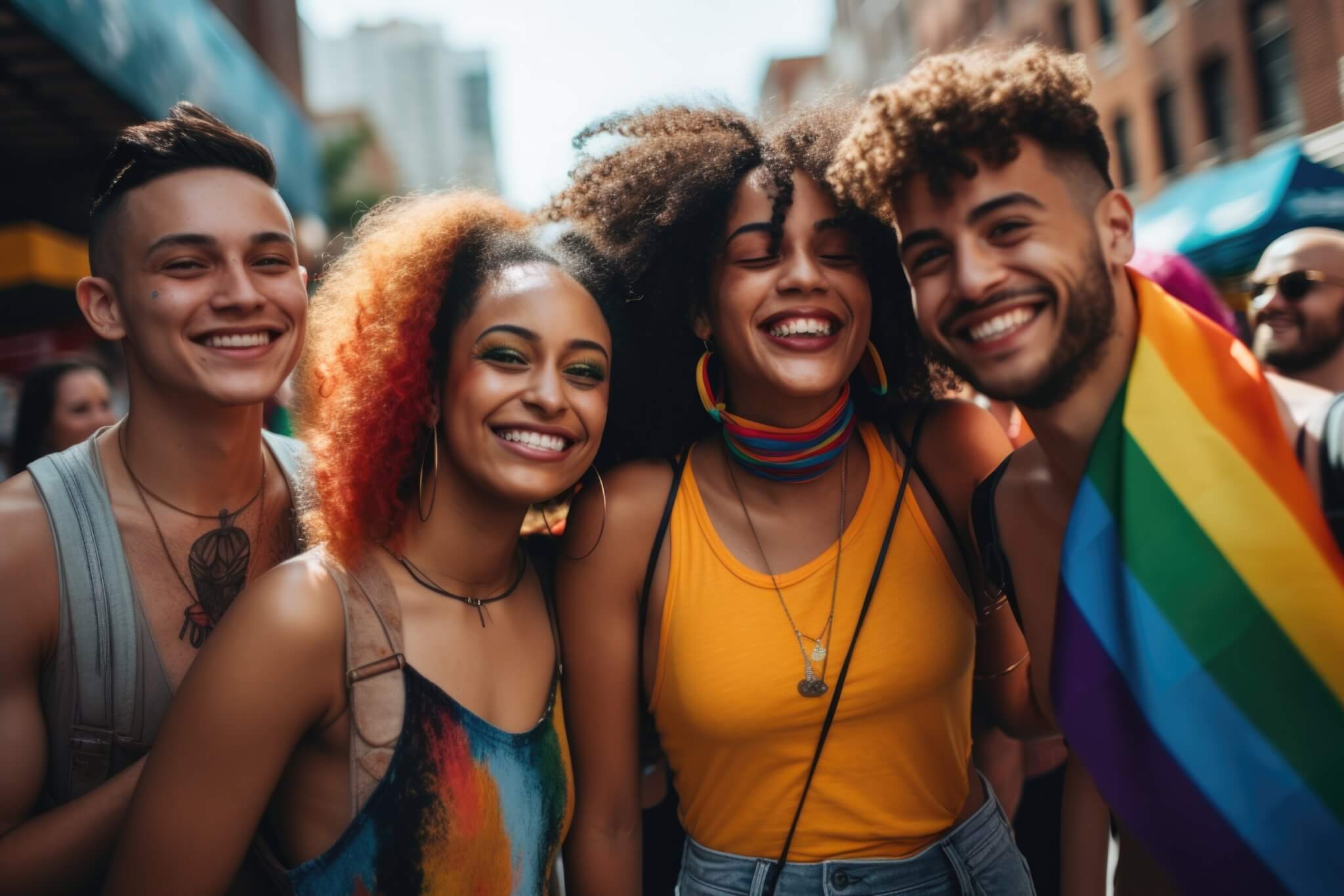
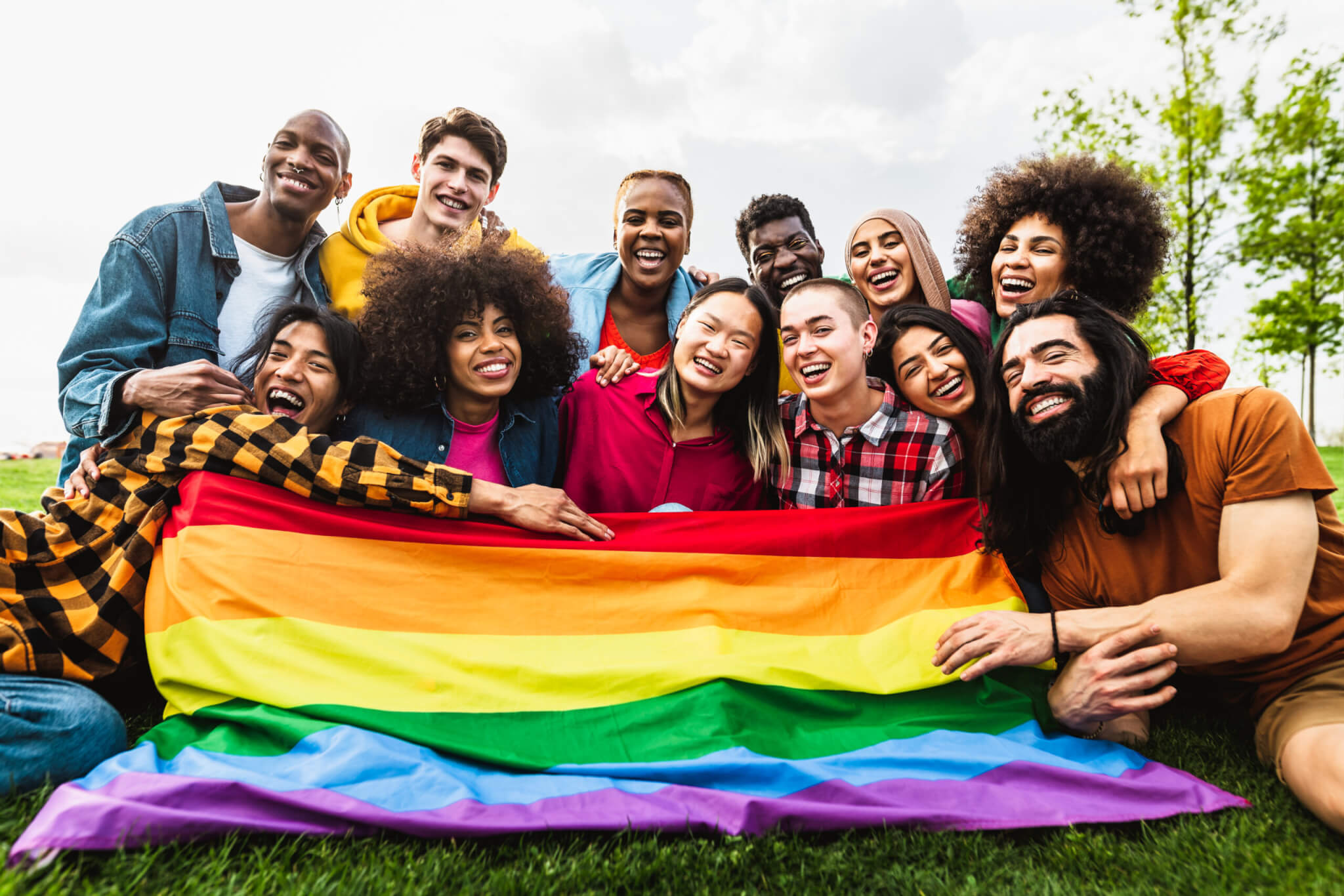
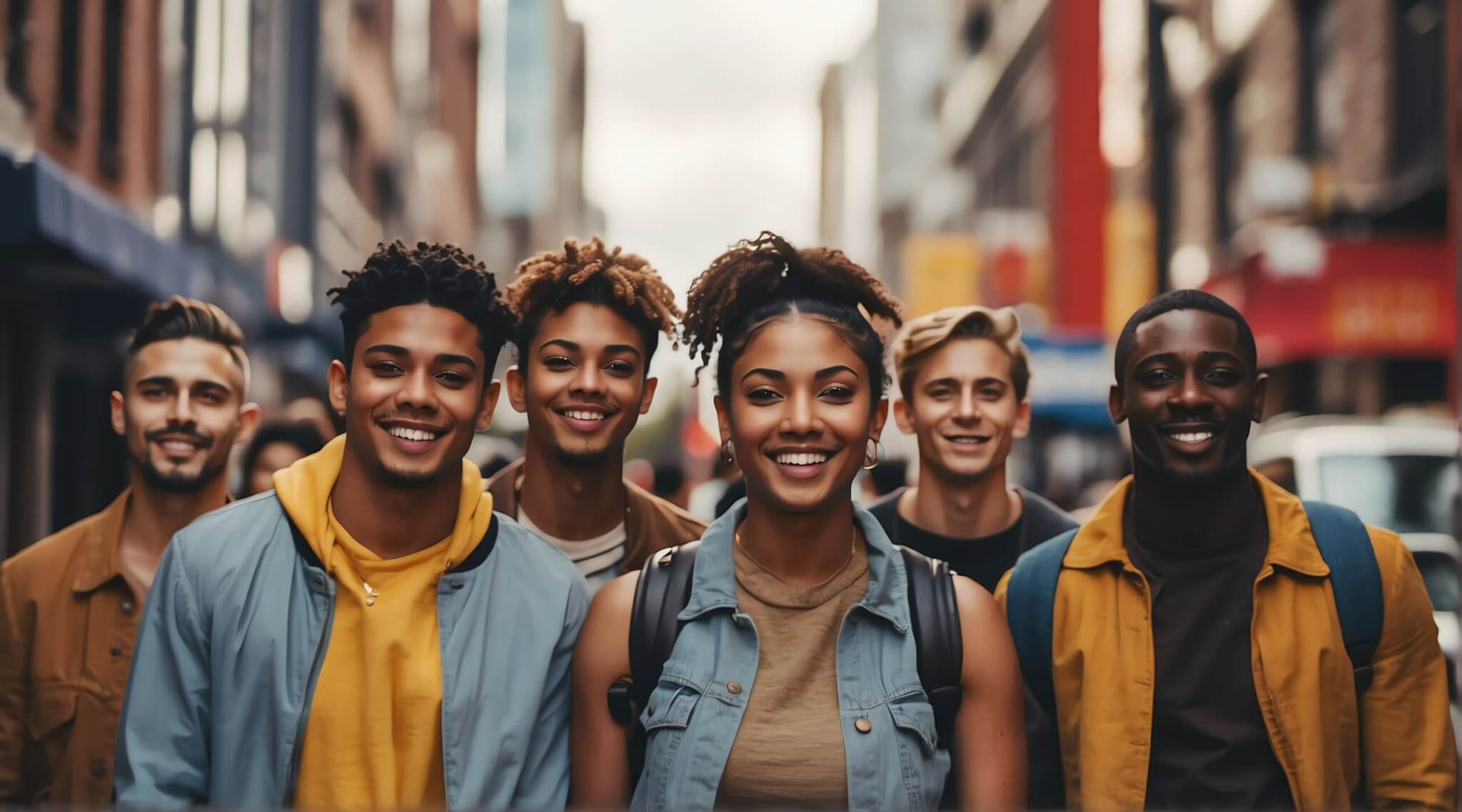
Trick question—the two on either end are.
It’s easy to see how representing “people” that aren’t any one person in particular can feel like a fantastic shortcut toward avoiding some common pitfalls for equitable storytelling. After all, you don’t need to get an imaginary person’s consent to be photographed. There are no worries about showing an image of a minor when that minor isn’t real. If you have data on the demographics of your community, you can set a query for those exact identity markers, and generate a host of body doubles that can stand in for people, with no concerns or barriers around privacy. And if you work alongside an at-risk or vulnerable group, you’re not at risk of exploiting anyone by using folks’ images for purposes that may feel extractive, like for fundraising materials or campaigns.
But using AI-generated images—especially in a sector that’s motivated by collective good rather than collective greed—is deeply inequitable. Here are two major reasons why.
AI flattens identity
Diversity in representation is key for celebrating difference, authentically depicting specific communities and peoples, and resisting historic norms of allowing any one identity to be the default for representation. When we use AI to generate images with a specific goal of representing diversity, we never succeed. This is because the technology behind how AI generates images is essentially incompatible with the actual definition of diversity. Artificial Intelligence creates images by learning from, referencing, and sampling all the existing images on the internet. The content already swirling around in every corner of the internet is the parent of the AI-generated image, and as such reflects and perpetuates the deep biases and history of inequitable power distribution that’s inherent to the internet.
The result is a flattening and genericization of identity, that creates a new, but all too human, form of stereotype. For example, the image below is an AI-generated image that Adobe Stock offered in response to the query, “LGBTQ Youth”. In this collection of individuals, there is an eerie sameness and monolithic quality from a community that’s historically been iconoclastic and celebrated for expanding society’s constructs around identity. They have similar smiles stretching across their faces; they’re all slim and able-bodied, their skin is mostly melanated, and their clothing even matches. When we ask a learning model—AI—to generate an image of any particular identity, we allow that technology to help define that identity—and in this case, it’s creating a pretty glossy representation of queerness.
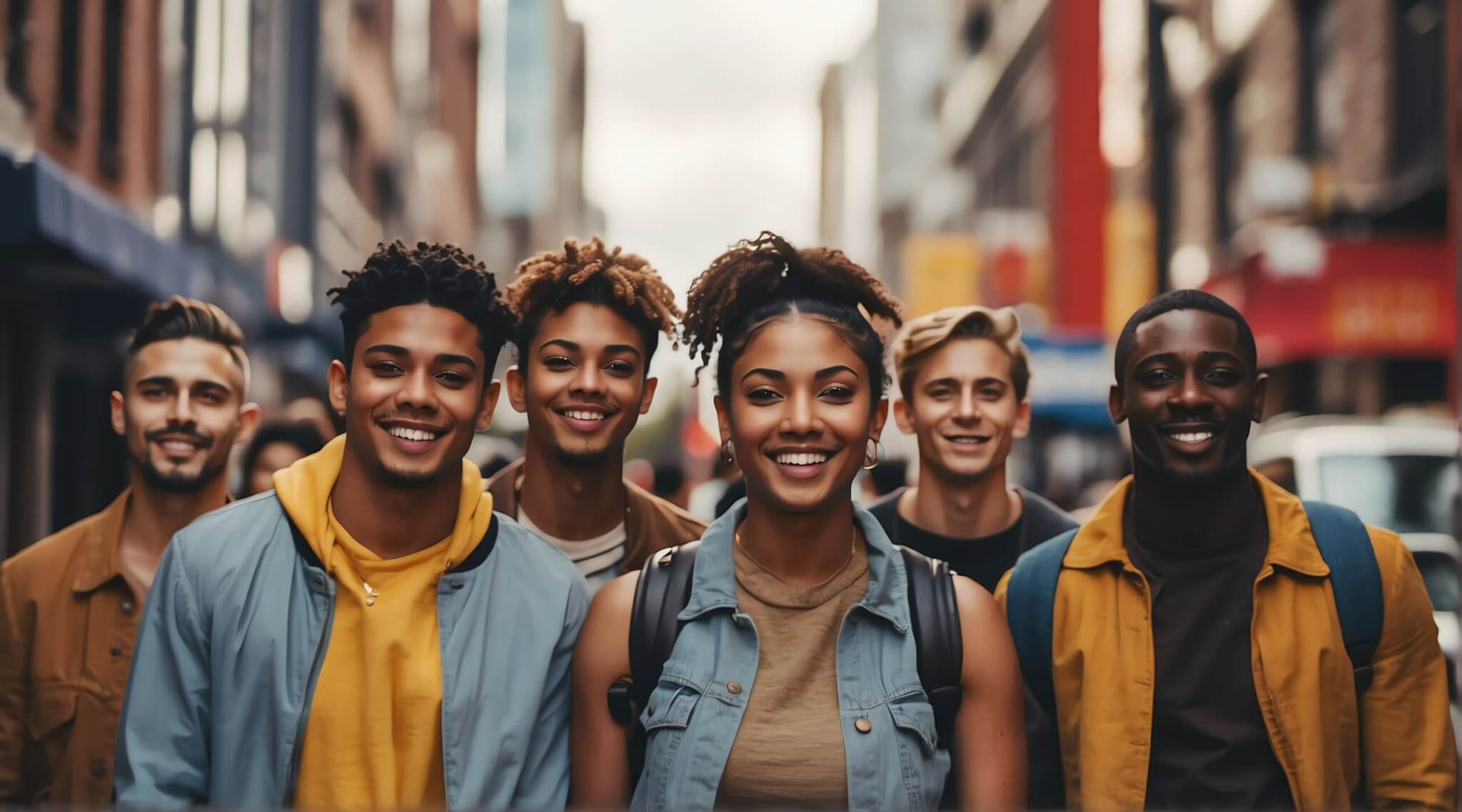
This is because the raw content that forms the DNA of these representations is an internet archive of data that underrepresents women, people of color, and marginalized communities, built by a tech sector notorious for lack of diversity and with an underdeveloped understanding of equity. Look, for example, at this image that celebrates Women’s History Month. I know that the AI learning model that created this isn’t a person, but I detect the male gaze.
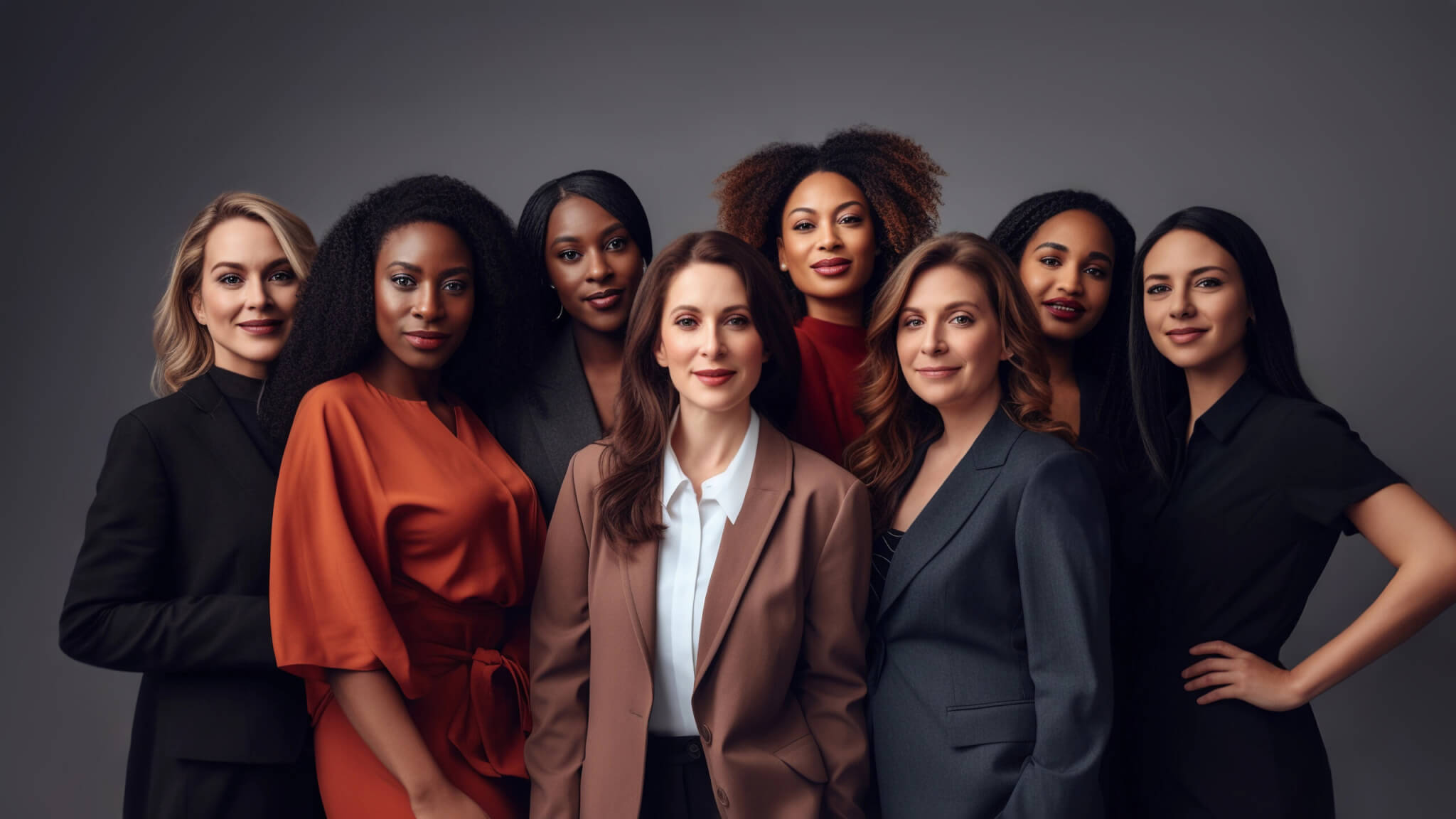
If these examples feel cartoonish and hardly like a threat to justice, this is because this technology, though advanced, is in its infancy. However, even very shortly when the technology is more refined, and visual signifiers are more nuanced, subtle, and difficult to discern, the underlying contradictions and incompatibilities with equity will remain. In short, depicting the diversity of your nonprofit’s community is a worthy goal. However, relying on AI-generated images to get there is not the road to authentic representation.
AI is at odds with equity
If we’re looking closely at how we use images to tell stories, and holding ourselves to a higher standard for consent around representation, then again, AI is not our friend. AI-generated content remixes and builds on millions of images found online, without compensating either the subjects or the artists who created them. Now it’s possible for a new and convoluted manifestation of harm around consent, that’s nearly impossible for anyone impacted to redress: instead of encountering a photo of themselves that they didn’t consent to and weren’t compensated for, a person might instead face a stereotypical and flattened representation of an identity that they hold, that reinforces false narratives, that was derived from a photo of themselves that they didn’t consent to and weren’t compensated for.
Additionally, AI-generated stock images are priced similarly to original content—but who’s benefiting from that money when there is no photographer to compensate? An equitable approach to authorship that advances inclusion involves moving toward a world where we’re commissioning local photographers and videographers from their regions and communities, who hold a nuanced understanding of cultural attitudes around privacy, consent, and representation. Allowing the learning model to author depictions of other cultures, genders, races, and more is not equitable and is frankly disrespectful to those populations.
This article unpacks just a few of our ethical concerns at the visual intersection of DEI and AI—there are many more that others are raising. AI is not monolithic—there are many different learning models, and it’s used in many ways and for nearly every type of work, including in the nonprofit and social impact spaces. And, we at Big Duck are actively discussing and exploring where and if we should consider incorporating these powerful tools. But when it comes to representing real people and real communities, especially in contexts where there’s a history of harm and unethical practices around telling stories, we’re steering clear.